©️ 2024 Kaiser Foundation Health Plan, Inc.
INTRODUCTION
Collecting high-quality data is essential for quality improvement, population health management and value-based care. [1][2][3][4] Performance measurement is the tool for monitoring and identifying opportunities to improve patient care. Consistently tracking and reporting on a standard set of quality measures will allow you to understand the current state of a patient population, identify any care gaps or health disparities, and monitor improvements over time.[5] Reporting on standardized measures is also used to monitor care across sites and align payment with achievement of quality.
Collecting and using racial and ethnic data allows practices to identify and respond to inequities and disparities in care, and is fundamental to population health management and to promoting health equity. High-quality data segmented by race and ethnicity can inform targeted performance improvements in access, continuity, preventive care and care management that ultimately lead to more equitable care and improved health for your patients.
These guides are designed to be helpful as part of an organized quality improvement strategy, with the goal of supporting substantive cultural, technological and process changes that improve population-based care. Enterprising practices can take on this work on their own with internal champions, including quality improvement, clinical and program leaders. They may be supported by practice facilitators, coaches or external consultants who help primary care practices improve population health management. The central content of the guide is organized into a sequenced set of evidence- or best practice-based key activities that, when applied to your local clinical context, can lead to improved ways of working. An onsite leader or champion can motivate peers and adapt the content in this guide for your setting, size, patient population and context.
In preparation for reporting, this guide offers steps and activities to ensure your practice is capable of reporting valid and reliable data for selected population health measures. The measures covered in this guide consist of seven Healthcare Effective Data and Information Set (HEDIS) measures designated as core measures by PHMI and 10 supplemental measures. All measures use standard HEDIS definitions and are aligned with DHCS Quality Strategy and Medi-Cal Managed Care Accountability Set and Alternative Payment Methodology (APM) 2.0. Using external HEDIS data to analyze patient populations can provide direction and focus on how to improve the health of your entire population, including those assigned but not yet seen.[6]
If your organization is interested in Going Deeper, additional content is available on using data from health information exchange and community information exchange platforms to create business intelligence tools that offer more robust, timely and holistic insights into service utilization and health outcomes. Finally, this guide covers data quality and reporting topics On the Horizon, such as using new technologies like remote patient monitoring and artificial intelligence to design, implement and optimize customized interventions to improve health for high-risk segments of the population.
HEDIS is a tool created by the National Committee for Quality Assurance (NCQA) to collect data about the quality of care and services provided by health plans. It consists of a standardized set of more than 90 healthcare performance measures across multiple domains. It is one of healthcare’s most widely used performance improvement tools. You can learn more about HEDIS from NCQA.
This guide is ideal for staff who will play a role in data governance and reporting within your practice. They might include:
- An executive sponsor.
- A clinical informatics or analytical staff member.
- A quality improvement coordinator.
- A clinical contact who can provide expertise on the clinical data feeds.
Regardless, all staff within the practice have a role to play in performance measurement. Part of the data governance and reporting team’s role will be to ensure that all staff understand the measures and protocols for data reporting, and that they follow through so that you’re able to report and use high-quality data.
Effective population health management requires that a practice’s data is accurate, reliable, comprehensive and accessible to care teams. Both your individual-level and population-level data should be:
- Accurate, reliable and comprehensive: Data should be high-quality and inclusive of demographic and social characteristics of your patient population. Validation and reconciliation processes will help you to achieve this.
- Tracked over time: Your practice should be able to track clinical measures over time. This is a demonstrated success factor for implementing population health management and patient-centered care. [7][8][9][10]
- Inclusive of all patients: Data should include the entire patient population— both those who have engaged in care (i.e., had a visit) and those who have been assigned to a practice by health plans but have not yet engaged in care. Accessing data and understanding the health needs of the entire patient population will require accessing external datasets as well as internal data.
- Visible to care teams: High-quality data should be visible to care teams at the point of care. This allows teams to more easily identify opportunities for improvement and to develop and monitor targeted interventions that address the care needs of the whole patient population the team is serving.[11] Additionally, having trusted, accurate population-level measures visible can engage and motivate providers and staff as they adopt a population-based perspective to care delivery for their patient panels.[12][13]
Key Activities
The key activities below will guide you through the process:
- Establish a data governance and reporting team.
- Understand the PHMI measures and specifications.
- Program and run measures.
- Assess internal data integrity.
- Identify and acquire external data.
- Conduct data validation and reconciliation.
- Report and use data to improve care.
After working through the key activities, you will be able to:
- Form a data governance and reporting team.
- Produce core quality measures using HEDIS specifications that have been adapted for PHMI.
- Develop and implement a data validation process that identifies gaps and solutions for improving data quality, such as reconciliation with managed care plans (MCPs).
- Produce quarterly core quality measures stratified by site and by race and ethnicity to identify and reduce disparities and improve performance.
Key Activity #1: Establish a data governance and reporting team.
Producing data that accurately reflects the care and services patients are receiving requires strong processes for data oversight, quality control and ensuring the data can be used throughout the practice (also known as data governance). A data governance and reporting team with clearly defined roles, responsibilities, systems and processes is critical to ensuring sustained high-quality data and reporting. A leadership structure should be established to oversee the work of developing policies and procedures that define and guide expectations for use of the electronic health record (EHR) system and the population health management platforms, and to ensure that they result in accurate and complete data to effectively manage your patient populations.
1. Determine which roles in your practice or larger organization are responsible for data governance.
Each organization may have different councils or committees already in place that meet data governance needs or could be adapted to include robust data governance. Larger organizations may need multiple dedicated layers of governance, but smaller ones could incorporate oversight into existing leadership committees and roles. Regardless of the size of an organization, the scope of governance is roughly equivalent, but the complexity and the roles of those engaged in data governance may vary.
At a minimum, the data governance and reporting team should include:
- An executive sponsor.
- A clinical informatics or analytical staff member.
- A quality improvement coordinator.
- A clinical contact who can provide expertise on the clinical data feeds.
Individuals in these roles become stewards of improving and ensuring the integrity of data collection, analysis and reporting. Their scope will include:
- Identifying data priorities that align with organizational priorities.
- Developing or updating data-related policies and procedures.
- Taking responsibility for the application of policies and procedures.
- Identifying and defining specific data-entry improvement opportunities
- Working with leadership and the clinic staff to address workflows for intake, registration, provider and care team staff documentation, and other applicable processes to ensure needed information is being obtained.
- Overseeing external data ingestion.
- Overseeing performance measurement analysis.
- Overseeing reporting performance results.
2. Determine the data governance strategy.
The scope of the data governance and reporting team includes establishing the basic framework that consists of the policies and procedures, standards, ownership, decision rights, roles and responsibilities, and data accountability as it relates to:
- Collection.
- Retention.
- Use.
- Accessibility.
- Sharing of healthcare data.
Practices will also need to determine which reporting system will be used to report each measure (e.g., within a data platform, regionally by an RAC or through a manual report development process).
Policies and procedures for EHR and population health management platforms can be expansive, touching all areas of an organization. At a minimum, they should
include the following key components, tailored to your data collection needs and EHR platform:
- A description of the appropriate use of EHR system documentation features and
capabilities. - A description of standards of conduct for program integrity of documentation
and how it will be included in staff and management training. - Health information management of any external informational content.
- Definition of role-based access to health record-related information.
- Policies and procedures on information acceptance, retention and formats.
- Attestation that quality requirements are aligned with latest DHCS Medi-Cal managed care targeted guides and requirements for primary care providers.
- Workflows to optimize data collection (intake, registration, documentation, etc.).
Key Activity #2: Understand the measures and specifications.
1. Review the core measures and supplemental measures.
PHMI aims to improve health outcomes and patient experience of care, provide better access to care, and increase health equity. To promote success in value-based reimbursement structures that support these goals, Performance measures were specifically chosen to align with measures in the APM measure set, as well as for
meaningful overlap with managed care plans’ (MCPs) Pay-for-Performance (P4P) measures. By working to understand and accurately report these measures now, practices will be better prepared to succeed in the MCP P4P programs, CA APM and other value-based payment structures.
The measures of focus include seven core measures and 10 supplemental measures, all from the HEDIS measure set.
Core HEDIS Measures for PHMI
PHMI Populations of Focus |
Measures |
---|---|
Children |
Child Immunization Status |
Well Child Visits in First 30 Months of Life |
|
Pregnant people |
Prenatal and Postpartum Care |
Adults with preventive care needs |
Colorectal Cancer Screening |
Adults living with chronic conditions |
Controlling High Blood Pressure |
Comprehensive Diabetes Care |
|
People with behavioral health conditions |
Depression Screening & Follow-Up for Adolescents and Adults |
Supplemental HEDIS Measures for PHMI
PHMI Populations of Focus |
Measures |
---|---|
Children |
Child and Adolescent Well Care Visits |
Immunization for Adolescents (Combo 2) |
|
Well Child Visits in First 30 Months of Life (15 to 30 months) |
|
Pregnant people |
Prenatal and Postpartum Care (Timeliness of Prenatal Care) |
Prenatal Depression Screening and Follow-Up
|
|
Postpartum Depression Screening and Follow-Up
|
|
Adults with preventive care needs |
Breast Cancer Screening |
Cervical Cancer Screening
|
|
People with chronic health conditions |
See “all adults” |
People with behavioral health conditions |
Depression Remission or Response for Adolescents and Adults |
All adults |
Adults’ Access to Preventive and Ambulatory Health Services |
The measures utilize HEDIS specifications, a standardized set of healthcare performance measures developed by NCQA, with certain modifications to make them relevant to providers. HEDIS measures are used by a wide variety of institutions and initiatives in the healthcare field, promoting industry-wide alignment and opportunities for benchmarking performance, including: APM, MCP P4P, California Department of Health Care Services’ Managed Care Accountability Set (MCAS), Center for Medicare and Medicaid Services (CMS) and 90% of America’s health plans.
For more about HEDIS measures, visit the NCQA HEDIS and Performance Measurement webpage or access the full list of HEDIS measures and brief descriptions for the HEDIS measurement year 2023.
While HEDIS measures are specified for health plans, many other healthcare entities adapt the measures for use in other settings, including at the provider level for programs like APM and provider P4P. This is because the industry-wide acceptance and broad use of HEDIS allows for comparisons across organizations and with national standards and benchmarks, and uniformity with measures used in other healthcare programs.
Health centers may find that HEDIS measurement is a shift from the more familiar Uniform Data System (UDS) measures that are routinely reported to the Health Resources and Services Administration (HRSA). One key difference is that HEDIS measures capture all patients who are assigned to the health center from a health plan, including those who have not had an encounter. In contrast, Uniform Data System measures capture only patients who have had an encounter at the health center within the year. Using HEDIS measures offers an examination of the population that the practice is responsible for and identifies opportunities for outreach, engagement and improvement in care. Understanding the importance of this new measurement set will require education across the organization.
All staff within the practice have a role to play in performance measurement, including office staff or medical assistants who schedule patients and enter information into the patient’s medical record; clinical staff who provide and document care and services; quality and analytics staff who produce and monitor the measures; and the leaders who oversee and determine the resources available for services and performance measurement.
Each staff member should have a strong understanding of:
- What is HEDIS performance measurement?
- Why is it being done?
- How does it contribute to patient care and the organization’s overall mission?
- What is their specific role in the process to ensure team buy-in and support and the fulfillment of responsibilities?
Creating understanding of the overall purpose of PHMI, its measures and their specifications requires a multipronged approach that tailors messaging and education to the type of staff.
2. Ensure all staff understand PHMI and measures.
All staff need to understand the purpose of PHMI, the purpose and function of reporting core measures, and their personal role in measurement and reporting. An all-staff training is an opportunity to inform and engage staff on their central role in measurement and performance. Training should include all staff and providers, and be understandable by a nontechnical audience. Data Quality and Reporting 101 is a PowerPoint deck developed for health centers and intended to support this kind of training in partnership with your practice coach and subject matter experts (SMEs).
Whether you use the deck provided by PHMI or one you design independently, the training content should include:
- Explanations of the overall purpose of PHMI and the measures to be reported.
- A plain language overview or “101” of HEDIS measures.
- The basics of measure specifications and reporting.
- An explanation of each performance measure and what the care team would need to provide and document to demonstrate performance.
The key functions critical to performance measures that are shared across staff, which should be covered in the training, include:
- Providing appropriate care in a timely manner.
- Recording accurate and complete patient demographic information in the medical record, including race and ethnicity.
- Recording accurate and complete documentation of care in the medical record, and properly coding the services.
- Ensuring documentation of information related to services provided outside the practice is reflected in the medical record (e.g., following up on specialist care).
3. Ensure data and quality leads have a detailed understanding of measures and specifications.
Staff responsible for data quality and reporting activities need a thorough understanding of the technical aspects of the core measures for PHMI, their specifications and reporting requirements. Accessing, understanding and accurately applying the measure specifications is important to ensuring alignment with other reporting efforts and having accurate data to monitor population health improvement efforts.
The Core Measure Specifications Manual was developed to provide a complete orientation to the technical aspects of PHMI measurement and reporting via the PHMI Data Reporting Tool (DRT). It is targeted toward a technical audience of quality and data analytics staff, and defines and describes the following:
- PHMI background and performance measure alignment.
- Reporting timelines and instructions.
- Understanding and interpreting HEDIS specifications and value sets.
- PHMI modifications to HEDIS reporting requirements.
- General guidelines for PHMI reporting and understanding specifications.
Convene staff responsible for data and quality reporting to orient them to the manual. Have these staff fully review measure specifications independently, and then reconvene to discuss issues of clarity as well as the data governance protocols that have been established to ensure timely, accurate data collection and performance measure monitoring and reporting.
Key Activity #3: Program and run measures.
Practices need to evaluate their capacity to produce and report core measures with fidelity to HEDIS specifications and adaptions made for PHMI. Start by examining the current reporting processes for core measures to determine what may need to change to ensure they can be accurately calculated and reported. You will need to look closely at how you are currently running or programming the measures and the resources being used in that process (e.g., EHR or population health management platform).
The Measure Calculation and Reporting for PHMI resource outlines how practices can develop a step-by-step process for assessing and improving capability to calculate and track the core measures. It includes a measure calculation checklist that you can use with the support of your practice coach and SMEs to identify and address any gaps in your data collection and calculation processes.
This resource outlines criteria for each core measure that can be used to:
- Identify current gaps in your ability to accurately calculate the denominator, exclusions, numerator and subpopulations.
- Identify steps to manually calculate the measure, if needed.
Key Activity #4: Assess internal data quality.
The utility of performance measures depends upon accurate data that reflects the care and services patients receive. Health centers participating in PHMI will need to report on HEDIS measures accurately and completely. After developing a strong grounding in the core measures, you’ll need to assess the quality of your internal data sources. Focus on building capacity to compile and extract all internal data needed for reporting, ensuring you have no data gaps. Best practices for internal data collection include strong documentation of all services received, maximized usage of structured data fields, and accurate and timely coding of services based on this documentation.
Data should be stored in structured fields according to a standard file layout so that it can be accurately captured from a patient’s medical record. This requires the availability of those fields within the medical record with capacity to store structured information, as well as consistent and accurate use of those fields by all providers of care.
If standard fields are not available or not used properly and consistently, the care and services provided may not be reflected in performance rates, and you may not be able to examine and report rates by subpopulations. Otherwise, a large-scale repeated effort to cull data from unstructured fields would be needed to accurately reflect the care patients are receiving. This could result in additional hours of staff time and reporting deadlines and performance payments can be negatively impacted.
In conjunction with your practice coach and SMEs, you’ll need to identify the standard data fields, define how information should be stored, assess current use of those fields, and define steps going forward to ensure standard capture of data by care providers. You can tailor this process based on your practice’s unique factors, such as the specific EHR you’re using. PHMI has produced two tools that will help you accomplish this work.
Standard Data Fields for HEDIS Measures can assist your practice in examining and improving your utilization of standard data fields. Use this tool to determine:
What data are needed?
- Is your practice utilizing all data sources adequately?
- Is information being captured and stored in inconsistent locations that would not be reflected in calculated rates?
For each core measure, the Documentation and Coding Playbook provides a description of the measure, assessment, documentation and coding standards needed to meet numerator compliance with PHMI specifications for the measure. It also supports accurate documentation efforts and delineates proper coding.
By using Standard Data Fields for HEDIS Measures in conjunction with the Documentation and Coding Playbook, your practice will be better able to understand and implement the assessment, documentation and coding that will meet the measure criteria. This is a starting point for quality improvement related to data capture.
Key Activity #5: Identify and acquire external data.
In many instances, HEDIS measures for PHMI will rely on external data or data that reflects care or services received outside of your organization. This may include outside laboratory tests, screenings, follow-up visits from a specialist (e.g., OB/GYN, endocrinologist or behavioral health), hospital data (e.g., admission data, discharge data and transfer data), or primary care rendered in other settings (e.g., urgent care center or at a different primary care provider’s office), and race and ethnicity data from managed care plans (MCPs).
To accurately report core measures, your practice will need to have access to these data, and have processes to ensure these data are properly stored within the EHR in ways that are accessible to data analytic platforms used for reporting. This could include coordinating with external providers to ensure ongoing communication and feedback, or connecting to the data via a third party, such as health information exchanges (HIE) or MCPs.
The External Data Acquisition resource informs processes for connecting with and utilizing different types of external data. It identifies potential external clinical data sources needed to report core measures and provides resources to assist in connecting to the data.
Key Activity #6: Conduct data validation and reconciliation.
Before reporting, measures must be validated. This means that each measure is verified to be an accurate, reliable reflection of the care that has been delivered or of the outcomes a patient has experienced. You should have a validation process in place to ensure the accuracy of measurement and reporting, which should include:
- A process for validation of internal data.
- An additional process to reconcile practice-produced core measures with MCP P4P reports where core measures are used.
- A process for ongoing reconciliation of practice and MCP data.
Process for Internal Data Validation
Data validation is the act of reviewing and confirming that data from your practice used to calculate PHMI core measures is of a minimum acceptable level of quality and accuracy. Depending on the analytics platform and process used to report each measure, validation can be an automated or a manual process or a combination of both. Having a systematic process with clear and specific steps to validate internal data will increase the level of confidence in the accuracy of the data reported for the core measures, and yield better decisions regarding performance improvement interventions and ongoing monitoring.
Data validation can occur at each step in the calculation process of a measure to ensure the accuracy of that specific component. Validation includes overall checks to confirm the final rate (e.g., do the numerator and denominator make sense?), reasonable breakdowns by subpopulations, and specific checks to confirm that a service or visit was correctly coded on a member level.
The Data Validation Process resource delineates an overall approach for basic data validation, describing key steps you can perform to validate your performance rates for PHMI core measures. These include general steps applicable across all measures (e.g., validating eligible population and stratified populations), as well as steps specific to each core measure, when relevant.
This data validation approach can be applied to other quality measures, including supplemental measures, other P4P measures, or as part of a broader data governance program.
Process for Validation and Reconciliation of MCP Data
Reconciliation against MCP rates is critical to the PHMI data validation process because:
- The MCPs are producing similar rates through their P4P programs for an overlapping subset of measures that offer an important comparison opportunity.
- It ensures MCP P4P rates are an accurate reflection of the care provided and outcomes achieved.
- It ensures practices have accurate and reliable rates for the pursuit of quality improvement.
The Validation and Reconciliation of MCP Data resource lays out a process to compare your practice and MCP measurement rates. For the PHMI measures that overlap with MCP P4P, practice rates are an opportunity to cross-check the validity of MCP rates and ensure optimal performance.
Reconciliation is a multistep process. To compare and analyze practice and MCP rates, it is important to know the steps, sources and criteria that the MCPs used to calculate their rates, which can be found in P4P manuals. You’ll need to identify any variation in measure calculation methodologies, such as differences in measure specifications and time frames for the reporting period, as well as any differences in determining the eligible population or subpopulations. Data from the MCP, such as patient-level detail or gaps in care reports, will be needed to facilitate reconciliation. The resource includes a checklist to assist in the reconciliation of the core measures, and you can work with your coach and SMEs to maximize the efficiency of engaging MCPs in tandem with other practices.
Key Activity #7: Report and use data to improve care reporting.
Improving care and achieving your specific quality goals requires developing and utilizing reporting mechanisms that provide relevant stakeholders with access to timely and relevant data.
The Measure Calculation and Reporting for PHMI tool provides best practices to assist with the tracking and reporting of core measures.
You should be able to produce reports with the following best practice features:
- Rates segmented by subpopulations, such as patient race and ethnicity, clinic site and provider.
- Year-to-date (YTD) rates, in addition to 12-month rolling measurement. While rolling measurement is used to enable practices to track improvement throughout the year, YTD rates align with health plans and APM, and will allow for intervention planning and additional tracking throughout the year.
- Comparisons to benchmarks.
- Comparisons to prior time periods.
- Comparisons to peers (for providers) or similar organizations.
- Patient level drill down.
- Comparison tracking with cascading goals, which calculate the number of patient services (gaps) needed to reach goals.
- Tests of statistical significance to determine if change over time and/or rates by subpopulations are significantly different.
- Realistic internal targets for continuous improvement.
Consider the various audiences who need access to calculated rates, on what cadence, and how the data could be best displayed for that audience. For example, organizational leadership and your board of directors may need formal presentations aligning with their meeting cadence; care teams should have frequent at-a-glance access through tools such as dashboards to proactively monitor care and identify opportunities for improvement.
For most audiences, presenting complex sets of data in a visual, pictorial format will allow for a greater understanding of concepts and help people identify patterns. Using such data visualizations is a reporting best practice. The most effective data visualizations use the most appropriate structure or format for the data and are very simple. Tables, Graphs, Dashboards, & Reports: Data Visualization Best Practices, produced by HealthData Viz, offers tips for creating effective tables and graphs used in dashboards for healthcare professionals. 10 Best Practices for Building Effective Dashboards, designed for Tableau users, includes data visualization best practices and examples that apply, regardless of the platform you’re using. Both tools can help you create data visualizations that are easy for others to access, read and understand.
As you utilize robust and ongoing reporting to monitor care and identify opportunities for improvement, you will be able to formulate strategies to act upon those opportunities to improve population health and close gaps in care. Following are ways you can share data and engage staff in the collaborative work of improving care:
- Post measurement rates in shared areas and remind staff during daily huddles to use the data to underscore the collective focus on quality improvement.
- Have care teams examine and refine their workflows to ensure screenings and other key activities are being conducted.
- Use the data to inform small tests (change workflows, tweak processes, etc.) to improve performance.
- Identify patterns of gaps in care and develop strategies to address them (e.g., improving care delivery for patient subpopulations, reeducating staff with lower rates, etc.).
- Compare rates by subpopulation (e.g., different race and ethnicity or clinic site) to determine discrepancies, and develop interventions to address health inequities and health disparities between groups.
Advancing Equity Through Data Quality and Reporting
Delivering quality care demands that we address the needs of subpopulations with attentiveness to their specific care needs and structural barriers to health. Even in organizations where performance on HEDIS and other quality measures is high, significant racial and other sociodemographic disparities often persist.
Strengthening population health requires going beyond aggregated HEDIS measures and identifying subpopulations that have lower rates of receiving certain types of care, and may require different forms of support. The process to get robust patient-level data is complicated and doesn’t have to be perfect to advance the work. Even imperfect data on race, ethnicity and language (REAL), sexual orientation and gender identity (SOGI), and social needs provide valuable insights to guide care delivery and coordination.
Practices can contribute to improving health and reducing disparities by considering the following approaches to strengthen data quality:
The initial step in addressing disparities is to collect high-quality data that fosters a comprehensive understanding of each patient. This entails incorporating REAL data, demographic data (age, gender) and social needs data. By leveraging this information, healthcare practices can gain valuable insights into disparities in access, continuity and health outcomes.
- This guide, developed by the American Hospital Association, provides care systems with guidance about collecting and using REAL data in meaningful ways to understand and address healthcare disparities: Reducing Health Care Disparities: Collection and Use of Race, Ethnicity and Language Data.
- This white paper from the Commission to End Health Care Disparities provides context and recommendations for collecting and using REAL data in ambulatory settings: Collecting and using race, ethnicity and language data in ambulatory settings.
- The Fenway Institute shares methods for gathering sexual orientation and gender identity (SOGI) data from patients in clinical settings: How to Gather Data on Sexual Orientation and Gender Identity in Clinical Settings.
- This training module by the American Medical Association (AMA) describes why collecting REAL data is important, and how to use this data to improve quality outcomes: Collecting Patient Data: Improving Health Equity in Your Practice.
- This presentation by the Community Health Care Association of New York outlines the importance of accurate and standardized REAL data and how to routinize data workflows: Race, Ethnicity and Language Data Collection.
As your data quality continues to improve, you can further tailor efforts to enhance healthcare specifically for subpopulations experiencing disparities.
- This brief by the American Hospital Association Center for Health Innovation provides guidance on how to use data to identify, investigate and intervene to address health disparities: Using Data to Reduce Health Disparities and Improve Health Equity.
- In this case study, Advancing Health Equity highlights how a health system in Rhode Island developed a phone-based care management intervention to address depression in Latinos, who typically receive less treatment and have poorer outcomes: Telephone-Based Depression Care Management.
- This framework developed by the American Hospital Association provides guidance for how healthcare systems can stratify REAL data for the use of identifying healthcare disparities: A framework for stratifying race, ethnicity and language data.
- In this publication, the American Heart Association highlights a pharmacist delivered care model for hypertension in barbershops to address disparities in blood pressure in Black men: Barbershop-Based Care Dramatically Trims Blood Pressure.
As you engage in the work, partnering with individuals with lived expertise in data sharing ensures the efforts are more responsive to the needs of community members, and the insights provide valuable context to inform data sharing. You may also find it helpful to partner with community organizations outside the healthcare practices to strengthen and broaden the data being utilized to guide clinical and programmatic decisions to address disparities.
- This brief from the Center for Health Care Strategies shares key lessons and insights to incorporate how the community perspective can improve data sharing efforts: A Community-Centered Approach to Data Sharing and Policy Change: Lessons for Advancing Health Equity.
- This practical guide from the California Health Care Foundation serves as a tool for communities considering cross-sector data sharing between health and homeless systems of care. It provides practical tips for some of the challenging components of cross-sector data sharing: How to Share Data: A Practical Guide for Health and Homeless Systems of Care.
Supporting Change
Effectively transitioning any complex change in an organization requires strong support from leadership and ongoing, clear and repeated communication that answers the questions: Why? What does this mean for me and my role? What are the new expectations?
For data quality and reporting specifically, there are several answers that you can share with those involved in the data and reporting role within your practice:
- Collecting high-quality data is essential for quality improvement, population health management and value-based care.
- Measuring performance will allow everyone within the practice to monitor and identify opportunities to improve patient care.
- Consistently tracking and reporting on a standard set of quality measures, especially disaggregated by race and ethnicity, will reveal the current state of a patient population, identify disparities in care and outcomes, and allow for monitoring of improvements over time.
As you work your way through this guide, and are thinking about the changes that will be required of your staff, map out what it specifically means for different people’s roles and responsibilities and the expectations for how and when those changes are made. Be sure to communicate this early and regularly, and engage leaders who can be ambassadors and reinforce a very consistent message.
In addition to ongoing, clear and repeated communication, having the sponsorship of a senior leader is absolutely crucial for the success of data governance projects and ensuring effective change.[14] A senior leader can help in the following ways:
- Bring credibility to the changes around data quality and reporting.
- Overcome the obstacle of organizational silos and encourage collaboration to meet data and reporting requirements.
- Serve as an advocate and problem solver by addressing potential roadblocks and ensuring data governance efforts remain on track.
- Demonstrate and communicate the value and impact of data governance initiatives to other senior leaders and staff.
- Secure ongoing resources, support and commitment at the highest levels of the organization.
Going Deeper
New approaches to both measuring and exchanging clinical information are key drivers for going deeper with data quality and reporting. Electronic clinical quality measures, also known as eCQMs, are measures specified in a standard electronic format that use data electronically extracted from health information systems, in contrast to the more typical approach of using claims data, data abstraction or chart review to compile population data.[15] For more information about the development and electronic specifications for eCQMs, visit the eCQI Resource Center. Electronic clinical quality measures offer the potential to save time through electronic measurement, enable access to real-time clinical data to drive quality improvement, and improve the accuracy and comprehensiveness of clinical data for value-based payment arrangements.[16]
Despite the potential for eCQMs to substantially improve data quality and reporting, there are technical, human and system-level challenges to surmount for successful implementation.[17] In a qualitative study about the implementation experience of smallto-medium primary care practices using eCQMs through the Agency for Healthcare Research and Quality EvidenceNOW initiative, practices report a number of key strategies for successfully adopting eCQMs for quality measurement and quality improvement.
Implementation suggestions include:
- Remain nimble about technological approaches, as native EMR functions relating to eCQMs may not be adequate to achieve reporting and quality improvement (QI) goals. Third-party services may be needed, and navigating these technical challenges requires a team with the appropriate resources and technical expertise.
- Be prepared to invest in strategies for eCQM data extraction, validation and reporting. This step not only improves data quality, but also creates an opportunity to engage providers and promote buy-in and acceptance of this approach to measurement.
- Consider deploying a practice facilitator with specific expertise in health information technology and eCQMs. Health information technology (IT) facilitators hold specific knowledge and capabilities for engaging primary care providers and teams on EMR and eCQM issues, which are generally beyond the scope of a typical QI-focused practice facilitator.
- Provide clinical feedback using eCQMs to primary care teams, including panel-level data, along with both local and national comparisons on the same eCQMs.[18]
In addition to adopting eCQMs, practices interested in going deeper with quality measurement for population health might consider participating in health and community information exchange platforms in order to create business intelligence tools offering more robust, timely and holistic insights into service utilization and health outcomes. Health information exchange refers to the electronic exchange of information across healthcare provider settings, while community information exchange refers to the electronic exchange of information between a multidisciplinary group of healthcare systems, social service providers and community-based organizations in order to improve community care planning.[19][20]
As organizations consider entering into value-based payment arrangements with increasing levels of risk for population-level outcomes, adequate and holistic data describing population health and social service utilization, health behaviors, and social conditions will be critical to predicting and managing both risk and health improvement strategies.[21] Information exchange is not only critical for building population-level health management capacity, but is also a key driver for addressing individual health concerns. Health information exchange can reduce duplication of services and improve patient safety and outcomes, while community information exchange platforms can enable healthcare organizations to more effectively make referrals to needed social services.[22][23]
As with any other technology adoption, implementation of health and community information exchanges can be rocky. To support successful engagement, facilitators can:
- Include adequate organizational participation and change leadership, end-user engagement and workflow improvement.
- Address privacy parameters and concerns.
- Conduct ongoing community-level partnership and collaboration to optimize impact.[24][25]
For California healthcare providers, participation in data exchange is newly mandated by California’s Health and Human Services Data Exchange Framework, a statewide data sharing agreement that will require information sharing by healthcare providers, government agencies and social service providers. With the goal of improving the provision of “safe, effective, whole-person care,” the Data Exchange Framework takes a phased approach to requiring organizations of different types and sizes to engage in systematic data exchange. Entities are required to sign the Data Exchange Framework by the end of 2023, and data exchange begins on or before January 31, 2024. Some organizations have until January 31, 2026, to begin exchanging information. More information is available on the California Center for Data Insights and Innovation website.
Voices From the Field
Jodi Pekkala, MPH
Jodi Pekkala is a researcher and consultant with over 15 years of experience in evaluation, quality improvement and practice transformation. She is a Patient-Centered Medical Home Certified Content Expert™ (PCMH CCE™) who assists primary care practices through the various stages of transformation and quality improvement using individualized on-site technical assistance and virtual and in-person learning events. As a certified HEDIS compliance auditor (CHCA), she led annual audits of health plans and possesses in-depth knowledge of HEDIS data collection, measurement and reporting processes.
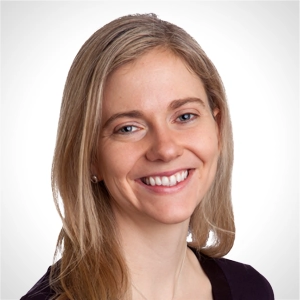
Q: What do you see as the current innovations in data quality and reporting?
A: Data quality and reporting is going towards digital quality measurement and using measures that pull data directly from the EMR rather than through supplemental files, claims or chart review. This is particularly important for measures that rely on clinical data. For example, metrics that focus not just on HbA1c process measures but the actual results of those tests. Using claims or supplemental files is messy, incomplete and potentially very labor intensive.
In digital quality measurement, we are able to pull data directly from the digital files, combine that information with data pulled from health information exchange platforms so that we’re able to access data from other providers, and see a more complete picture of a person’s care experience and health. The greatest impact for this innovation lies in not only having much easier access to complete data, but also using it in an interactive way with dashboards and drill-down reports to really understand what is happening with different segments of the population.
There is a real strategic opportunity with all of this high-quality, timely information to look at population risk levels, stratify the highest risk segments, and use that information to address gaps in care and design interventions accordingly. Ideally, you’re able to do it through these sophisticated platforms that allow for interactive dashboards, but there are also more accessible ways to do it, depending on the resources available to your practice. For example, using Excel spreadsheets, if that’s what’s available. This is really about understanding how to look at your population as a whole and systematically deploying your resources to maximize impact for improvement.
Q: What do you find particularly exciting or interesting relating to data, quality and reporting for advancing health equity right now?
A: Having better access to race, ethnicity and language (REAL) and sexual orientation and gender identity (SOGI) data, to be able to analyze population data for disparities. Of course, it’s not just about monitoring, but then dedicating resources to address disparities. Data quality and reporting isn’t innovation, it’s just that critical first step to identifying where your disparities lie and doing something about it.
Q: What are the new technologies that you are excited about for data quality and reporting?
A: There are emerging opportunities to use artificial intelligence to assess and target patient risk levels. For instance, with mammograms, there are changing general guidelines, but there are much more specific guidelines that can be rendered based on additional and specific information about individual people and populations. In the future, we’ll be able to use artificial intelligence to get much more targeted guidelines, rather than relying on generalities that are much more appropriate for some populations than others.
Remote patient monitoring is another new and exciting input for patient-level data, although there are some significant implementation challenges to utilizing it in the most effective way. Remote patient monitoring requires a lot of tech literacy and engagement on the part of the patient population, which is more challenging to overcome than figuring out how to distribute the technology itself. Another challenge with this technology is getting the data to the provider at point of care, which is much more complex than getting the data from the patient’s device back to the vendor. A specific example where we can see remote patient monitoring working well is through the CenteringPregnancy program, where there are new efforts to support women to take their own blood pressure measurements at home and then use telehealth to increase rural access to care.[26]
Q: What do you see as the biggest challenges for data quality and reporting?
A: There are enormous challenges to understanding and using the data at the provider level. It’s a culture shift, and a hard one to make when you have more patients than slots available.
One significant aspect of this challenge is getting people to accept that these data are true. People look at their rates and say, “That’s not accurate. I know. I provide that care.” You have to have a deep understanding of your data and what goes into it; what is in the numerator and the denominator. You really need to understand the minutiae of the measure. If the numerator requires compliance within two years, if you do it in two years plus one day, you don’t get the credit. If the parent refuses, you don’t get credit. You have to really understand what’s in and what’s out. For example, if there is a vaccine allergy, you can get credit, but it needs to be documented in the chart. You need to measure quality and you need to improve quality, because you are getting paid on quality. You need to get more laser focused on your interventions and measurement of improved outcomes. We all recognize that the outcomes are harder to measure and assess, but that is where the system is headed.
On the Horizon
Organizations interested in leading-edge developments in data quality and reporting should consider the implications of new technology for both collecting and analyzing data. As recognition grows about the impact of home and community life on patient health, so does interest in supporting patients to monitor and improve health in their home environments.
Remote patient monitoring (RPM) is a form of telehealth that uses technology to enable patients to collect and share physiological data, such as blood pressure and blood glucose levels, and behavioral data, such as medication adherence and physical activity, from wherever they are.[27] This type of remote patient monitoring can improve care management programs for chronic conditions and enable healthcare teams to intervene early when a health problem is beginning to escalate for a participating patient. Systematic reviews show promising results for the effectiveness of remote patient monitoring for supporting patients with common chronic conditions, such as diabetes, hypertension, chronic obstructive pulmonary disorder and cardiovascular disease, such as congestive heart failure. [28]
Remote patient monitoring outcomes can vary and key elements to optimize effectiveness include:
- Accurately identifying and engaging populations at high risk, especially those with high or rising risk for hospitalization.
- Effectively identifying a decline in health, which can be more difficult for some conditions than others. Even in the cases where acute changes to health status are hard to detect, RPM can be used to monitor longitudinal progression of illness.
- Ensuring timely and responsive care by creating automated or user-friendly data sharing processes for patients, and ensuring that care teams are providing ongoing monitoring and rapid responses with bidirectional communication for any issues that arise.
- Providing personalized care, including collaboratively designing RPM approaches with patients, offering personal and repeated patient training on RPM devices, as well as establishing personal thresholds for alerts to the monitoring team, which may vary from patient to team.
- Combining RPM with self-management support, including patient education and coaching to improve knowledge, and skills and behaviors to improve self-monitoring and management.
- Offering collaborative and coordinated care, which includes both patients and families or caregivers, multidisciplinary representation from the patient’s care team in both primary care and specialty care, and proactive facilitation of not just monitoring, but enhanced partnership between patients and providers.
RPM is likely to be most effective when understood as a new model of care, not just a new technology. This understanding requires thinking carefully about collaboratively designing the approach with all stakeholders, implementing and refining workflows, committing adequate resources to patient education and self-management support, as well as ongoing, bidirectional communication between patients and monitoring teams.[29] From an equity standpoint, while RPM and other forms of telehealth may be useful to patients who have barriers to accessing clinic-based care, there may be additional obstacles to engaging diverse patients in RPM effectively, from low digital or health literacy challenges to language and cost barriers. Culturally congruent or contextualized care with wraparound support for RPM, such as community health workers supporting patient adoption, may mitigate some of these challenges.[30]
Although medical professionals have been looking for ways to leverage artificial intelligence (AI) since the 1950s, advances to AI in healthcare have exploded in recent years with enormous growth in both technologies and research.[31] Under the umbrella descriptor of artificial intelligence, there exist a range of technologies with varying relevance to enhanced data quality and reporting for population health.[32] Although AI has a very broad range of implications for both public health and healthcare delivery, potential applications for primary care data quality and reporting include integrating AI into remote patient monitoring, risk prediction and intervention, and risk-adjusted empanelment.[33] The most relevant and immediate application of AI for population health in community health settings may be enhancing risk identification and prediction using medical, behavioral and social health data to create a more holistic picture of both individual and population-level risk characteristics.[34][35]
Although AI holds promise for enhancing healthcare delivery and population health, the technology also has the potential to reinforce and worsen health disparities. AI technologies that rely on existing datasets to learn and build functionalities will recapitulate biases that are present in the initial data, and may then be capable of replicating such biases at enormous scale.[36] Organizations developing or investing in AI should be careful to consider health equity implications for new technologies, as well as advocating for regulatory standards to mitigate the risk of reinforcing existing biases.[37]
TOOLS AND RESOURCES
SUGGESTED CITATION AND ACKNOWLEDGEMENTS
Recommended citation: Population Health Management Initiative. Jodi Pekkala, David Wedemeyer and Ariel Singer. Data Quality and Reporting Implementation Guide. In: Coleman K and Singer A, editors. Building the Foundation Implementation Guide Series. 1st ed. Oakland, CA: Kaiser Permanente, Health Management Associates, and Pyramid Communications; 2023.
Acknowledgments: This document was created by the Population Health Management Initiative (PHMI), a California collaboration of the Department of Health Care Services (DHCS), Kaiser Permanente and community health centers. We gratefully acknowledge the contributions of these partners and the Regional Associations of California (RAC) along with the many other academic, health plan and consulting partners who shared their expertise and insights freely as we collaboratively designed the initiative and created these guides.
Individual reviewers and content contributors to the first edition of the Building the Foundation Implementation Guide Series: Jennifer Sayles and Elise Pomerance (KP PHMI); Palav Barbaria, Yoshi Laing, David Tian, Jeff Norris and Karen Mark (DHCS); Allie Budenz and Cindy Keltner (CPCA); Jeanene Smith, Catherine Knox, Nancy Kamp, Ethan Norris, Leslie Brooks and MaryEllen Mathis (Health Management Associates); Anne Tillery, Sarah Starr, Karis Cady, Candace Jackson, Josh Daniel and Denise Rhiner (Pyramid Communications); and Veenu Aulakh.
With additional contributions from: Pyramid design team and Kaiser Permanente PHMI team.
Endnotes
- Mitri J, Gabbay R. Understanding Population Health Through Diabetes Population Management. Endocrinol Metab Clin North Am. 2016;45(4):933-42.
- Powell A, Rushmer R, Davies H. Effective quality improvement: some necessary conditions. British Journal of Healthcare Management. 2009;15(2):62-8.
- Rodriguez HP, Ivey SL, Raffetto BJ, Vaughn J, Knox M, Hanley HR, et al. As good as it gets? Managing risks of cardiovascular disease in California’s top-performing physician organizations. Jt Comm J Qual Patient Saf. 2014;40(4):148-58.
- Wise CG, Alexander JA, Green LA, Cohen GR, Koster CR. Journey toward a patient centered medical home: readiness for change in primary care practices. Milbank Q. 2011;89(3):399-424.
- Geboers H, Grol R, van den Bosch W, van den Hoogen H, Mokkink H, van Montfort P, et al. A model for continuous quality improvement in small scale practices. Qual Health Care. 1999;8(1):43-8.
- Rodriguez HP, Ivey SL, Raffetto BJ, Vaughn J, Knox M, Hanley HR, et al. As good as it gets? Managing risks of cardiovascular disease in California’s top-performing physician organizations. Jt Comm J Qual Patient Saf. 2014;40(4):148-58.
- Geboers H, Grol R, van den Bosch W, van den Hoogen H, Mokkink H, van Montfort P, et al. A model for continuous quality improvement in small scale practices. Qual Health Care. 1999;8(1):43-8.
- Rodriguez HP, Ivey SL, Raffetto BJ, Vaughn J, Knox M, Hanley HR, et al. As good as it gets? Managing risks of cardiovascular disease in California’s top-performing physician organizations. Jt Comm J Qual Patient Saf. 2014;40(4):148-58.
- Solberg LI, Stuck LH, Crain AL, Tillema JO, Flottemesch TJ, Whitebird RR, et al. Organizational factors and change strategies associated with medical home transformation. Am J Med Qual. 2015;30(4):337-44.
- Wise CG, Alexander JA, Green LA, Cohen GR, Koster CR. Journey toward a patient centered medical home: readiness for change in primary care practices. Milbank Q. 2011;89(3):399-424.
- Matthews MR, Miller C, Stroebel RJ, Bunkers KS. Making the Paradigm Shift from Siloed Population Health Management to an Enterprise-Wide Approach. Popul Health Manag. 2017;20(4):255-61.
- Powell A, Rushmer R, Davies H. Effective quality improvement: some necessary conditions. British Journal of Healthcare Management. 2009;15(2):62-8.
- Rodriguez HP, Ivey SL, Raffetto BJ, Vaughn J, Knox M, Hanley HR, et al. As good as it gets? Managing risks of cardiovascular disease in California’s top-performing physician organizations. Jt Comm J Qual Patient Saf. 2014;40(4):148-58.
- DataGalaxy. Powering Data Governance: Executive Sponsorship and Change Management [September 11, 2023]. Available from: https://www.datagalaxy.com/en/blog/powering-data-governance-executive-sponsorship-and-changemanagement/.
- eCQI Resource Center. Get started with eCQMs. Washington, DC: US Department of Health and Human Services; 2023 [September 11, 2023]. Available from: https://ecqi.healthit.gov/ecqms.
- eCQI Resource Center. Electronic clinical quality measures basics (eCQM 101) Washington, DC: US Department of Health and Human Services; [September 11, 2023]. Available from: https://ecqi.healthit.gov/sites/default/files/eCQM-Basics-508.pdf
- McClure RC, Macumber CL, Skapik JL, Smith AM. Igniting Harmonized Digital Clinical Quality Measurement through Terminology, CQL, and FHIR. Appl Clin Inform. 2020;11(1):23-33.
- Richardson JE, Rasmussen LV, Dorr DA, Sirkin JT, Shelley D, Rivera A, et al. Generating and Reporting Electronic Clinical Quality Measures from Electronic Health Records: Strategies from EvidenceNOW Cooperatives. Appl Clin Inform. 2022;13(2):485-94.
- Eden KB, Totten AM, Kassakian SZ, Gorman PN, McDonagh MS, Devine B, et al. Barriers and facilitators to exchanging health information: a systematic review. Int J Med Inform. 2016;88:44-51.
- CIE San Diego. What is CIE? San Diego: 211 San Diego; [September 11, 2023]. Available from: https://ciesandiego.org/what-is-cie/.
- Vest JR, Harle CA, Schleyer T, Dixon BE, Grannis SJ, Halverson PK, et al. Getting from here to there: health IT needs for population health. Am J Manag Care. 2016;22(12):827-9.
- Menachemi N, Rahurkar S, Harle CA, Vest JR. The benefits of health information exchange: an updated systematic review. J Am Med Inform Assoc. 2018;25(9):1259-65.
- Kryzer E, Nolan CM. Community Health Improvement: Social Care Is Healthcare. Front Health Serv Manage. 2022;39(2):17-26.
- Eden KB, Totten AM, Kassakian SZ, Gorman PN, McDonagh MS, Devine B, et al. Barriers and facilitators to exchanging health information: a systematic review. Int J Med Inform. 2016;88:44-51.
- Cartier Y, Fichtenberg C, Gottlieb LM. Implementing Community Resource Referral Technology: Facilitators And Barriers Described By Early Adopters. Health Aff (Millwood). 2020;39(4):662-9.
- Runkle JD, Sugg MM, McCrory S, Coulson CC. Examining the Feasibility of Smart Blood Pressure Home Monitoring: Advancing Remote Prenatal Care in Rural Appalachia. Telemed Rep. 2021;2(1):125-34.
- Thomas EE, Taylor ML, Banbury A, Snoswell CL, Haydon HM, Gallegos Rejas VM, et al. Factors influencing the effectiveness of remote patient monitoring interventions: a realist review. BMJ Open. 2021;11(8):e051844.
- Leo DG, Buckley BJR, Chowdhury M, Harrison SL, Isanejad M, Lip GYH, et al. Interactive Remote Patient Monitoring Devices for Managing Chronic Health Conditions: Systematic Review and Meta-analysis. J Med Internet Res. 2022;24(11):e35508.
- Taylor ML, Thomas EE, Snoswell CL, Smith AC, Caffery LJ. Does remote patient monitoring reduce acute care use? A systematic review. BMJ Open. 2021;11(3):e040232.
- Lawrence K, Singh N, Jonassen Z, Groom LL, Alfaro Arias V, Mandal S, et al. Operational Implementation of Remote Patient Monitoring Within a Large Ambulatory Health System: Multimethod Qualitative Case Study. JMIR Hum Factors. 2023;10:e45166.
- Secinaro S, Calandra D, Secinaro A, Muthurangu V, Biancone P. The role of artificial intelligence in healthcare: a structured literature review. BMC Med Inform Decis Mak. 2021;21(1):125
- Lavigne M, Mussa F, Creatore MI, Hoffman SJ, Buckeridge DL. A population health perspective on artificial intelligence. Healthc Manage Forum. 2019;32(4):173-7
- Lin SY, Mahoney MR, Sinsky CA. Ten Ways Artificial Intelligence Will Transform Primary Care. J Gen Intern Med. 2019;34(8):1626-30.
- Jiang LY, Liu XC, Nejatian NP, Nasir-Moin M, Wang D, Abidin A, et al. Health system-scale language models are all-purpose prediction engines. Nature. 2023;619(7969):357-62.
- Carroll NW, Jones A, Burkard T, Lulias C, Severson K, Posa T. Improving risk stratification using AI and social determinants of health. Am J Manag Care. 2022;28(11):582-7.
- Matheny ME, Whicher D, Thadaney Israni S. Artificial Intelligence in Health Care: A Report From the National Academy of Medicine. JAMA. 2020;323(6):509-10.
- Thomasian NM, Eickhoff C, Adashi EY. Advancing health equity with artificial intelligence. J Public Health Policy. 2021;42(4):602-11.